摘要:锂电池荷电状态(SOC)观测技术作为电池管理系统(BMS)的关键技术,在维持电池系统设备安全高效运作、延长电池组整体生命周期等方面均起着不可或缺的作用.本文以改善锂电池荷电状态的观测结果为目的,对锂离子电池荷电状态的观测方法进行了研究,基于二阶变参数锂电池模型,设计了一种有效的改善SOC观测精度的方法.首先,根据SOC的定义,建立了安时积分估计(AH),通过引入二阶变参数锂电池模型建立扩展卡尔曼滤波估计器(EKF),然后结合Takagi-Sugeno模糊模型原理,设计Takagi-Sugeno和EKF联合估计器(TS–EKF).最后,在Simulink仿真平台上验证了SOC观测方法的准确性和实用性.结果表明,本文所设计的Takagi-Sugeno和EKF联合估计器可以改善SOC观测精度.
关键词:荷电状态估计;二阶变参数锂电池模型;扩展卡尔曼滤波估计器;Takagi-Sugeno模糊;Takagi-Sugeno和EKF联合估计器
1 Introduction
With high energy density,long life period and low self-discharge rate,the lithium ion battery is known as one of the fastest growing and promising large scale energy storage battery in the field of power generation.However,thecoreproblemssuchassafety,performance and the lifetime of the battery have not been fundamentally solved.As one of the core technologies of bat-tery management system(BMS),the observation technology of the battery state of charge(SOC)plays an indispensable role in maintaining the safety and high Efficiency of the battery system and prolonging the battery’s life period etc.Accurate SOC estimation of battery can avoid system failures and prevent battery overcharge and over-discharge,which may cause permanent damage to the internal structure of the battery[1–2].However,SOC cannot be measured directly but must be estimated according to measurable parameters such as voltage and current.
Because of the necessity of SOC observation,A large number of SOC estimation methods have recently been proposed in the literature,these methods are divided into the following four categories:Amper-hour integral(AH)estimator method,look-up table method,data driven method and model based observation method.The AH integral estimator has been widely used due to its low computational cost and easy implementation.However,The error caused by environmentalnoise,current drift,initial value inaccuracy cannot be effectively eliminated and compensated by the method.Errors from current sensors also accumulate in the calculation process[3–4];The look-up table method is simple and easy to use,especially suitable for off-line operation.But this method is not sufficient for online realtime estimation and it also requires periodic correction of open circuit voltage(OCV)or electrochemical impedance spectroscopy(EIS)[5–8].The same as AH integral estimator method the battery must be tested under different current rates in advance.Data-driven approach has distinct advantages in solving system nonlinear problems,but this method requires collecting a large amount of experimental data for model training and consuming huge amounts of hardware resources;If the data used does not fully reflect the characteristics of the battery,then the SOC estimation error will be very large[9–13];Among all the SOC estimation methods,the model based observation seem to be the most practical choice for online SOC estimation at present.And because it has the characteristics of closed-loop feedback,the model observation method has good robustness for the estimation of the battery SOC[14–17].The main difficulty of the model based observation is the real-time accurate identification of model parameters.How to coordinate the contradiction between model accuracy and robustness so that it not only ensure high observation accuracy,but also can adapt to the battery cycle conditions and other internal state(such as health state of health,SOH)changes[18].This will be a question that deserves further study.
As mentioned in the previous described,model based observation relies on an accurate battery model for accurate SOC estimation.However,the internal parameters of the battery change during the charging and discharging process.It is difficult to establish enough accurate models to describe the external characteristics of all batteries.Therefore,in this paper,a new SOC observation algorithm—Takagi-Sugeno and extended Kalman filter union estimator(TS–EKF)union estimator,is developed by combining the model based and the data driven methods.The research ideas and results in this paper can be used as a reference to the multi-state joint estimation of the Li-ion battery for a deeper level.
In order to realize the observation method of SOC of the Li-ion battery,based on the conclusion of these basic tests and the commonly used and improved equivalent circuit model,the 2nd-order RC model with variable parameters is built to adapt to complicated and changeable working conditions;based on the battery model,an accurate and efficient SOC observation method is designed for Li-ion battery.Firstly,the AH integral estimator is built according to the definition of SOC,and then the EKF estimator is established by introducing the Extended Kalman Filter principle;Then,combined with the TS fuzzy principle,the TS–EKF union estimator is eventually designed.Finally,the accuracy and the practicability of the SOC observation method with the core technologies are verified based on the simulation platform of Simulink.
2 Battery model
The general nonlinear model(GNL)takes into account the activation polarization,ohmic polarization and concentration polarization of the lithium battery.In addition,most of the circuit elements in the GNL model are not constants,but are functions of voltage,temperature,or SOC.In order to satisfy the accuracy requirement of the battery,as shown in Fig.1,a 2nd-order RC model with variable parameters is chosen.This model is improved on the basis of the GNL model,two RC networks and one resistor,while removing the parasitic branch because the self-discharge rate of lithium battery compared to other types of batteries are low.

Fig.1 The 2nd-order RC model with variable parameters
In the 2nd-order RC model with variable parameters,the value ofRTis a variable parameter that is affected by the temperature of the lithium battery.The polarization resistance and polarization capacitance in the model change with the direction of the current.Where RbdandRpdarerespectivelystandforthedischargeac-tivation polarization and concentration polarization resistance;RbcandRpcare the activation polarization and concentration polarization resistance in the charging process respectively;CbdandCpdrespectively stand for discharge activation polarization and concentration polarization capacitance;CbcandCpcare the activation polarization and concentration polarization capacitance in the charging process respectively.The open circuit voltageUOCis a function of the lithium battery SOC,and the relationship between the two is fixed for the same cell.Ubatis the terminal voltage of the lithium battery,Ibatis the current of the lithium battery,thedischargeispositive,andthechargeisnegative.
based on the 2nd-order RC model with variable parameters and the Kirchhoff’s law of voltage and current,we can obtain the state space expression of lithium battery.The state equation and output equation are as shown in Eq.(1)and Eq.(2)[14–16]respectively.
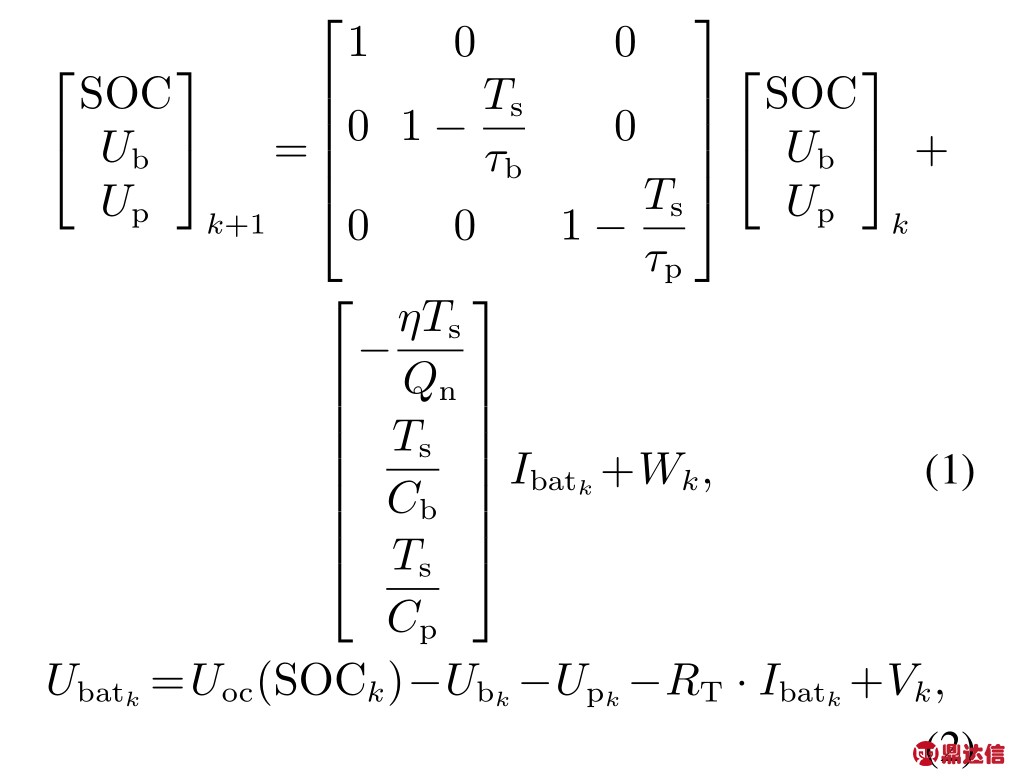
whereηstand for coulombic Efficiency.TheWkand Vkrespectively indicate the process and measurement noise.Tsis the model’s sampling time.Where τb stand for voltage response time constant of battery’s activation polarization.And the value ofτbis product of polarization resistanceRband polarization capacitance Cb.Whereτpstand for voltage response time constant of battery’s concentration polarization.And the value ofτpis product of polarization resistanceRpand polarization capacitanceCp.WhereUbandUpare the polarization voltage of activation and concentration polarization.Wk∼N(0,Q),Vk∼N(0,R).
The least squares method is used for identification of 18650GA lithium battery,the following relations can be obtained:

whereIindicates battery’s charging and discharging current.
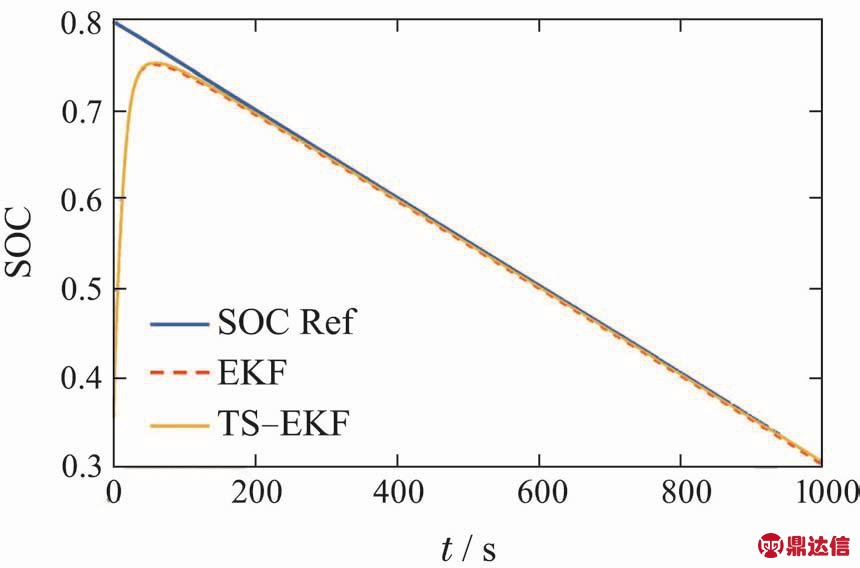
whereTindicates the ambient temperature.
The results of the other parameters are shown in Table 1.
Table 1 Other parameters in battery model
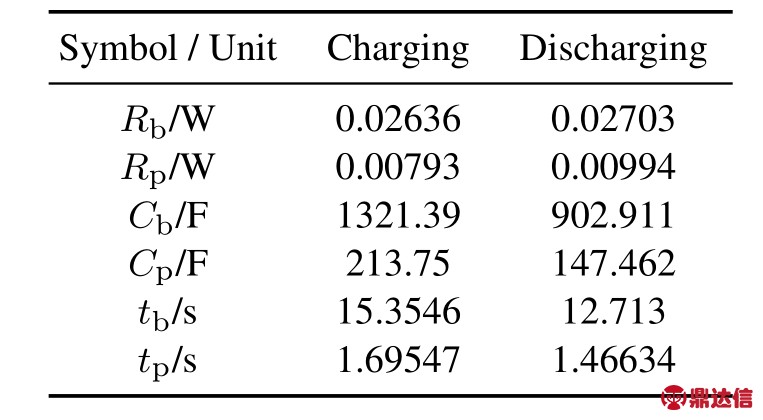
The 2nd-order RC model with variable parameters can better describe the static and dynamic response characteristicsoflithiumbattery,takingintoaccountthe model calculation speed and accuracy.Therefore,based on this model,the SOC observation method for lithium battery will be developed.
2.1 Model verification
The Sanyo NCR–18650GA type Li-ion battery,shown in Fig.2(a),is used as the experimental research object.It has the characteristics of long cycle life,stable performance,high safety and large capacity.It is generally used in electric vehicle power system.The charging and discharging experiments of target batteries were carried out by using NEWARE’s tester BTS–5V20A(A maximum 20 A charge or discharge current with 0.1%of full scale accuracy),the connection diagram of BTS–5V20A battery tester is shown in Fig.2(b).
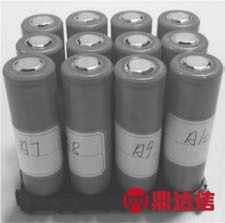
Fig.2(a)The Sanyo NCR–18650GA Li-ion battery
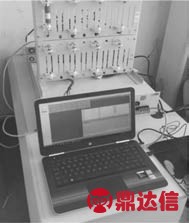
Fig.2(b)The BTS–5V20A battery tester
The hybrid pulse power characteristics(HPPC)test is used to obtain the voltage response of the battery.A current pulse signal was applied to the HPPC test at a total of 9 test points with a SOC of 0.1,0.2,···,0.9.In each selected SOC test point,the battery is first discharged for 10 s at a current rate of 5C(ie,16.75 A),then left for 30 s,and then the battery is charged for 10 s at a rate of 5C.The current pulse duration is 50 s.The HPPC current pulse signal is shown in Fig.3.
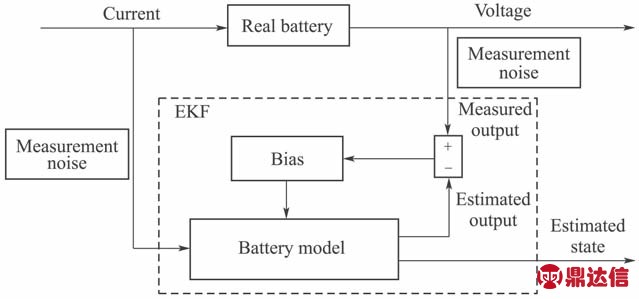
Fig.3 HPPC current pulse signal
The current excitation in the HPPC test is input into the simulation model of battery.The feedback voltage of discharge and charging for each SOC point are compared with the actual voltage corresponding data.The comparison results are shown in Fig.4 and Fig.5 respectively.In the comparison curves of the discharge section and charge section,the SOC corresponding to each group of curves is 0.1,0.2, ···,0.9.Because there’re too many curves in the graph,the SOC corresponding to each curve is not shown one by one.
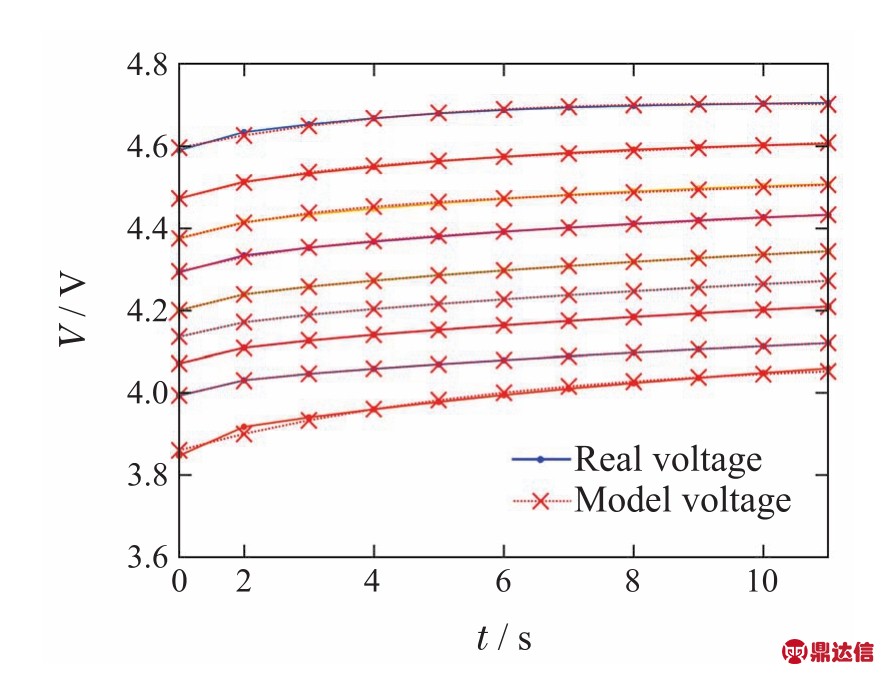
Fig.4 The comparison curves of the charge
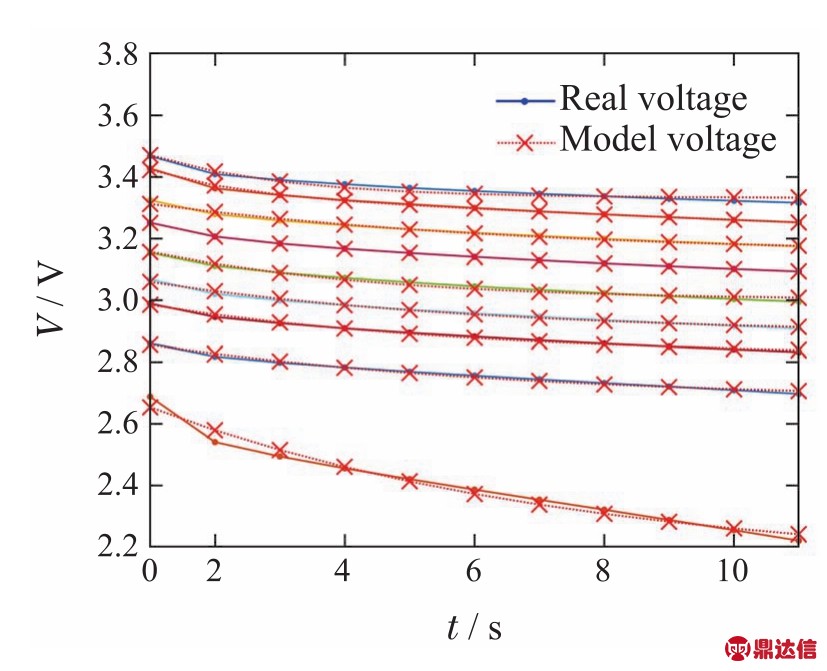
Fig.5 The comparison curves of the discharge
It can be seen from Fig.4 and Fig.5 that the voltage data of battery at the test points(SOC equal to 0.2∼0.9)are close to the actual data except for the test points at SOC equal to 0.1.When SOC is 0.1,the internal concentration polarization has a great in fluence on the lithium battery,which leads to the increase of the error.After calculation,the error square deviation of each group of data is less than1× 10−4.Therefore,the model has reliable accuracy.
3 Desgin of SOC observation method
In this section,an accurate and efficient SOC observation method is designed for Li-ion battery based on 2nd-order RC model with variable parameters.
3.1 Amper-Hour(AH)integral estimator
In general,the amount of electricity used in a lithium battery is expressed by an integral of the discharge currentIbatfor a period of time.The expression for the calculation of the electric energyCis as follows:

After discretization,the discrete expression of the AH integral method can be obtained as follows[3–4]:

whereSOCkis SOC of battery at timek,SOCk+1is SOC of battery at timek+1.The difference between SOC at two times is the amount of electricity released withintof the sampling interval.Obviously,the accuracy of SOC estimation is related to the accuracy of the current sensor and the size of the sampling interval∆t.Inaddition,inthechargeordischargeprocessoflithium battery,battery capacityQnwill change with the health of SOH,it changes depending on the cell cycle and other conditions,including the current change curve and ambient temperature.In practical,the cycle conditions of the battery are usually uncontrollable.The abovementioned problem is the limitation of using the AH integral method as a means of battery SOC observation.
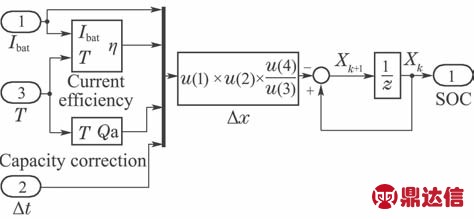
Fig.6 MATLAB/Simulink model of AH integrator estimator
The MATLAB/Simulink model of the AH integrator estimator is shown in Fig.6.The real-time current of the lithium battery measured by the current sensor is used as the input of the AH integrator estimator,and the real-time SOC of the lithium battery is calculated as the output of the AH integrator by accumulating the change of lithium battery current.In addition,the temperature correction module is introduced for the battery rated capacityQn,and the temperature and current correction modules are introduced for the coulombic Efficiencyη.
3.2 Extended Kalman filter(EKF)estimator
The extended Kalman filter is one of the most celebrated and popular data fusion algorithms in the field of information and processing[19–21].The principle and structure of EKF method for observing lithium battery SOC is shown in Fig.7.The Current and voltage of the battery with measurement noise and battery temperature are the input of EKF estimator.The EKF estimator’s output is the estimated SOC.EKF algorithm module can effectively filter measurement noise and system disturbance,and the bias between the measured output and the model output is used to constantly modify the model state,eventually making SOC estimation value approaching the true state.
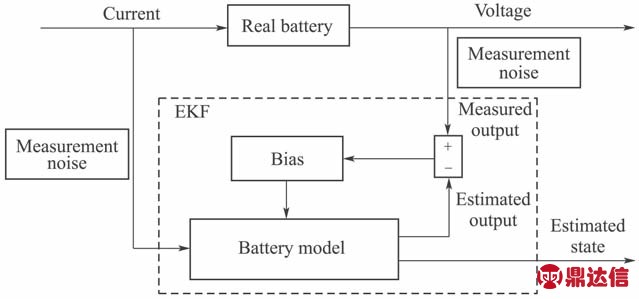
Fig.7 The structure of EKF estimator
The nonlinear part should be added to the system for a Li-ion battery model,the nonlinear system could be described as follows:
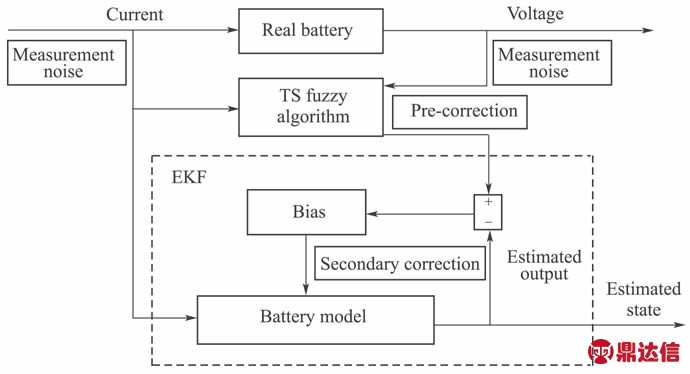
where W(k)and V(k)are independent white noises.
based on the state equations in Eq.(8),the key design flow of the corresponding EKF method can be divided into the following five steps:
1)Initialize the state estimate Xoldand the error covariance Pold.
2)Calculate the system matrix A,the prior state estimate Xudand the prior noise covariance estimator Pudfor the target system at present.The formula is as follows:
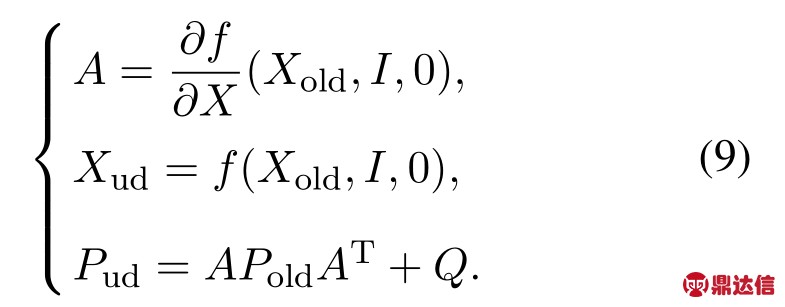
3)Calculate the system output matrix C.based on Eq.(9),Calculate the Kalman gain K.The formula is as follows:
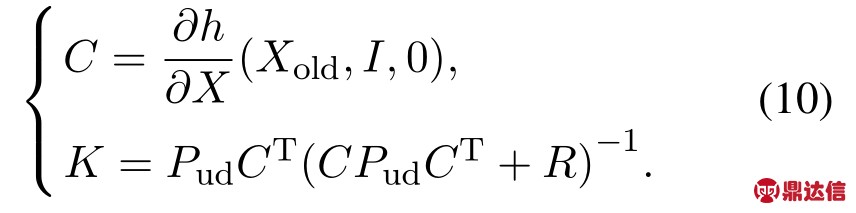
4)Calculate the system output Ynew,based on output equation in Eq.(8).Adjust state estimator Xud and Pud,according to the Kalman gain in Eq.(10)and the difference between the output measurement Ymeasureand the system output Ynew:
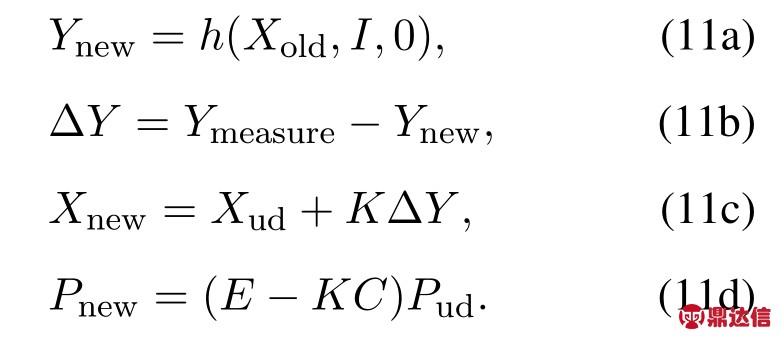
5)Update the state estimate Xoldand the error covariance Pold:
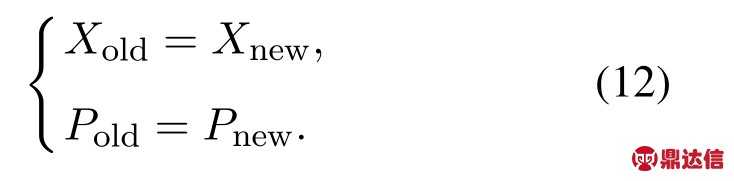
It is worth noting that we need to give the extended Kalman filter parameters—SOC(0),Ub(0),Up(0),P(0)noise covariance matrix Q and P—to the appropriate initial value in order to obtain better filtering estimation effect and faster convergence speed.In this paper,SOC(0)is set to the final SOC value stored in the memory.Ub(0),Up(0)is set to zero.And the P(0)is initialize to unit matrix.The Q matrix is set to zero;the R matrix is set as the square root matrix of the deviation caused by the measurement equipment(such as voltage,current,temperature sensor,etc.).The EKF estimator flow chart is shown in Fig.8.
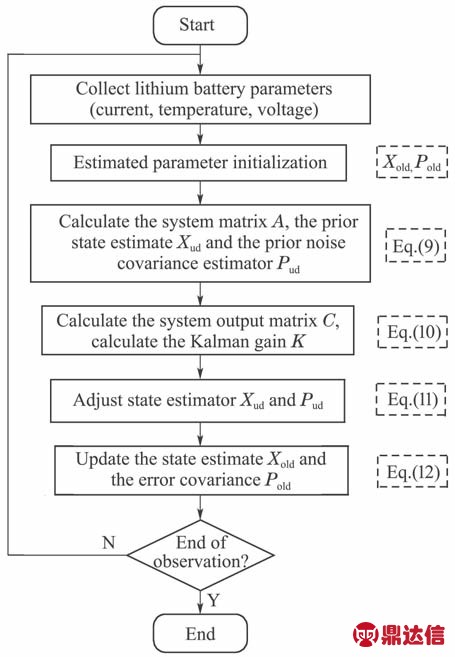
Fig.8 The flow chart of EKF estimator
3.3 The TS–EKF union estimator
Takagi-Sugeno fuzzy models formulate as a set of rules[22–23]:
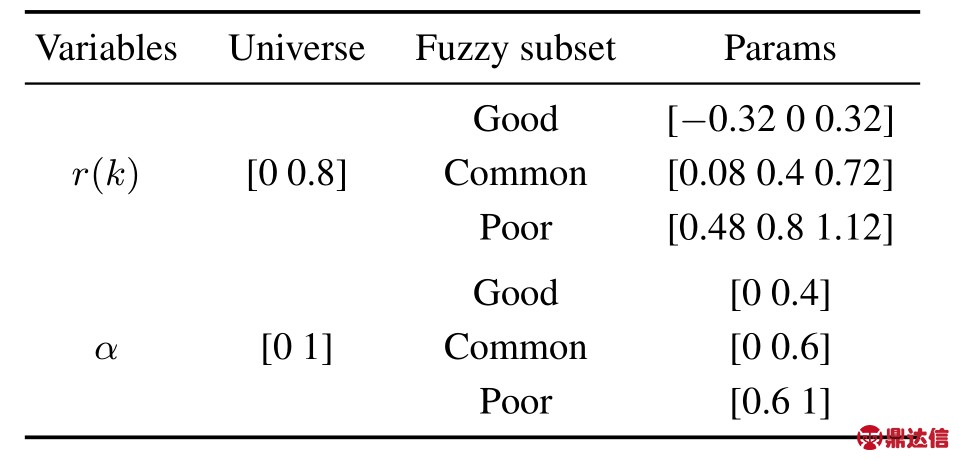
Where c is the number of fuzzy rules,n is the input variables number of the TS fuzzy model,x1(k),x2(k),···,xn(k)are the regressive variables consisting of out put and in put data at the kthinstanceandbefore,are the membership functions associated with the ith rule,yi(k+1)is the ith output component of the system,
are the consequent parameters of the submodel(fuzzy rule)i.
Denotesβias the fitnessgrade of submodeli,thus the model output y(k+1)at the(k+1)th instance can be calculated by the following equation:
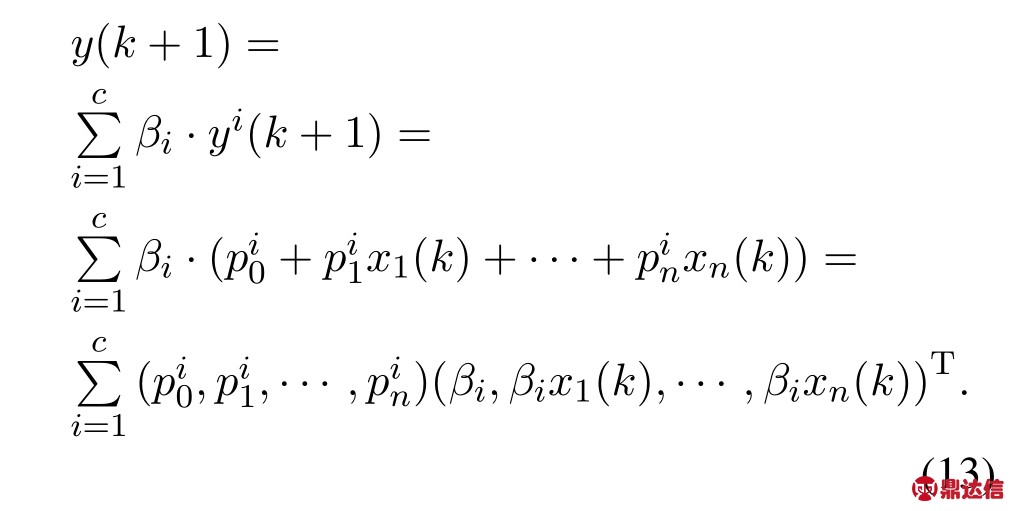
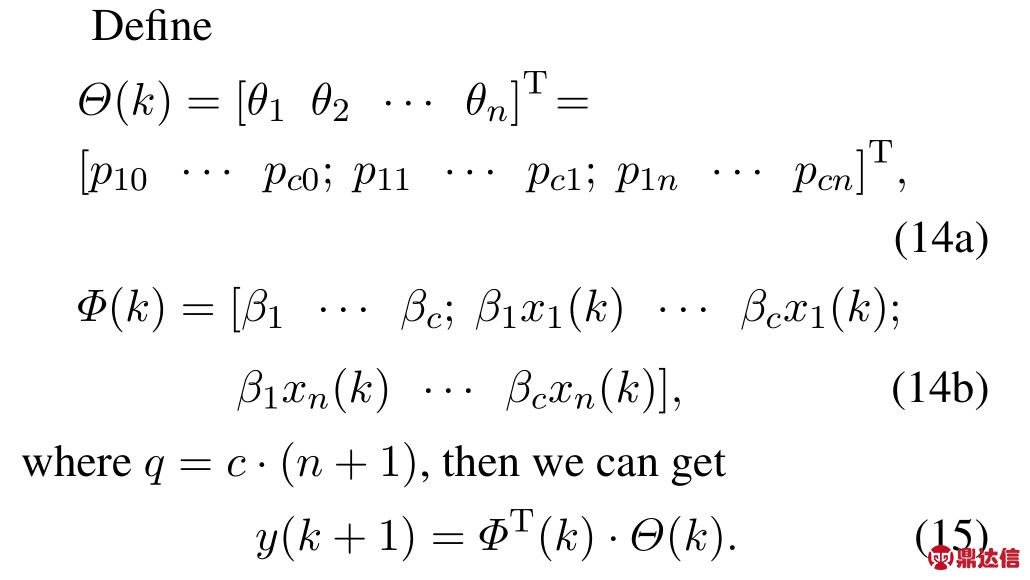
There are many online identification methods of TS fuzzy models[3–4,11,24–27],in which a method called online recursive identification of TS model is used in[4].Although the test results show that the method has reliable accuracy,its Efficiency is too low,is not suitable for the application of lithium batteries in the SOC observation system.In order to balance the accuracy and fastness of the TS fuzzy algorithm,this paper adopts the weighted recursive least squares method to calculate and adjust the parameters of the algorithm module.
The design method of the online TS fuzzy algorithm module is as follows:
1) Initialize all of parameters of TS fuzzy model,including number of fuzzy rule c(c>1),Least squares weighted regression covariance matrix Γ(1)= ΩE,the consequent parameters of fuzzy model Θ(1)=0,the cluster center vector

the fuzzy rule study ratio λ(0< λ <1),the fuzzy factor m(m>1).
2)Read the input variable x(k)and calculate the distance between the x(k)and each(k−1)th instant cluster center vi(k−1)from the following equation:

3)Estimate the membership degree of x(k)according to each(k−1)th instant cluster center vi(k−1):

4)Adjust the cluster center vector V(k−1)based on the membership degree and fuzzy rule study ratio λ at the(k−1)th instant:

5)Update the distance and the membership degree of x(k)according to the new cluster center vec-tor V(k):
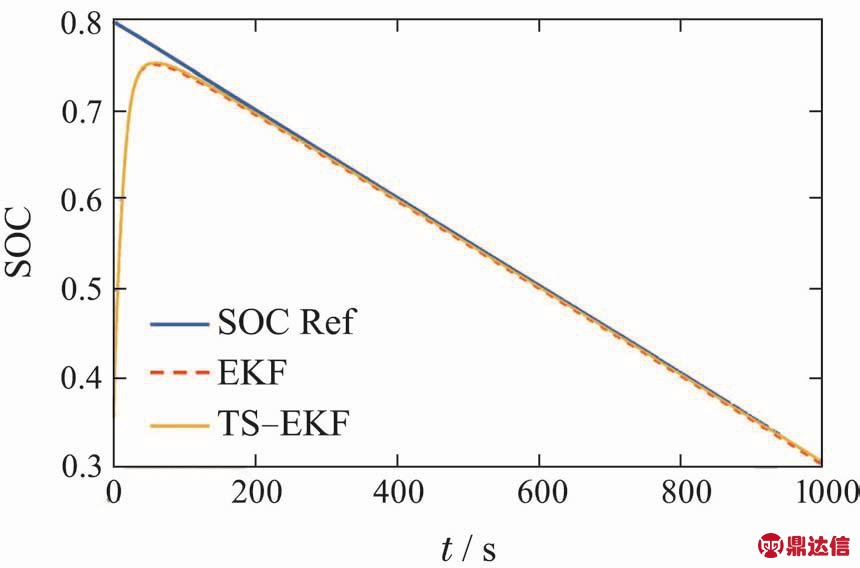
6)Calculate the fitness grade βiof the ith submodel with respect to x(k):

7)Determine the consequent parameters Θ(k)by the weighted recursive least squares method and update the covariance matrix Γ(k).


8)Finally,The predictive output can be derived from Eqs.(13)–(15).
9)Let k=k+1,and return to Step 2,until the control process end.
Combined with EKF estimator and TS fuzzy algorithm module,the TS–EKF union estimator was established to realize accurate observation of lithium battery SOC.The structure of the TS–EKF union estimator is shown in Fig.9.
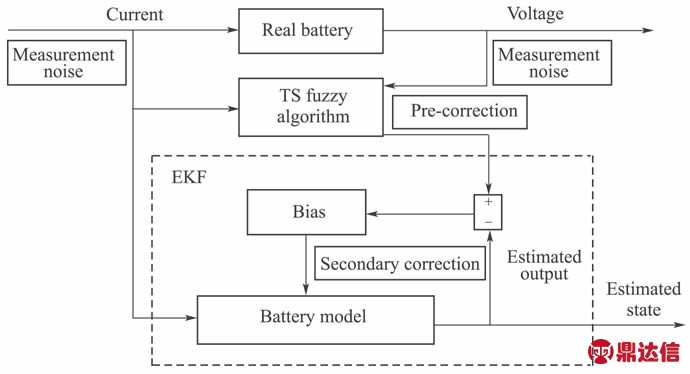
Fig.9 The structure of TS–EKF union estimator
Talbe 2 lists the parameters of the TS fuzzy algorithm.
Table 2 Parameters of TS fuzzy algorithm
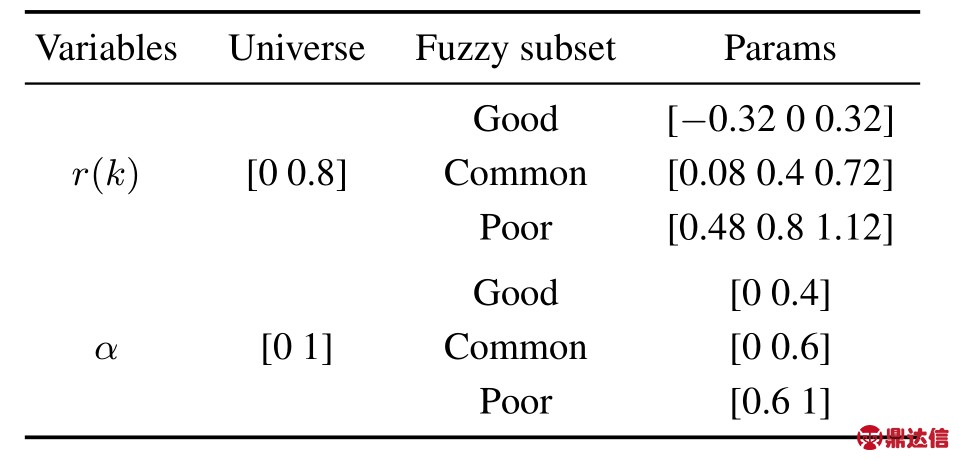
The output matching degree is defined as

Adjust the output according to the matching degree:

where 0<α<1 is the adjusting factor,and output is dynamically adjusted according to different r(k).In TS fuzzy algorithm,r(k)is taken as input and α is taken as output.
The working principle of the TS–EKF union estimator is as follows:Firstly,the TS fuzzy algorithm module is used to pre correct the input and output signals with measurement noise to suppress the effect of unknown random noise on output of the results.Then,the pre-corrected signal is input to EKF for the secondary correction.Finally,the bias between the output value of the TS fuzzy algorithm and the estimated output are used to quickly correct the lithium battery model,and the accurate observation of the lithium battery SOC is obtained.
4 SOC observation simulation comparsion
In this section,the proposed TS–EKF union estimator is compared with the AH estimator and the EKF estimator for the SOC observations on the Simulink platform.
4.1 Comparison of TS–EKF and AH Estimator
In the Simulink platform,the current excitation is input to the AH integral estimator and the TS–EKF union estimator for calculating the lithium battery SOC using the given test conditions.The observation results are shown in Fig.10.Among them,the SOC reference value is based on lithium battery test instrument(BTS–5V20A),so the reference value can be regarded as SOC real value.
Lithium battery is fully charged,that is,the initial state of SOC is 1.In the experiment,the initial SOC of the AH estimator is set to 1,and the initial state vector of the TS–EKF estimator is set to[0.9,0,0].A disturbance signal is added to the current input in the experiment.As shown in Fig.10,the AH estimator can be used to observe the SOC of lithium batteries,buttheobservationerrorwillincreaseastimegoeson.When the SOC of lithium battery drops to 0.1,the error of AH estimator has increased to 0.04,and there is a tendency to continue to increase.On the contrary,the TS–EKF union estimator is biased in the initial state,still can quickly converge to the reference SOC,and the steady state error of observation can be maintained within 0.01,which can meet the requirements of practical applications.
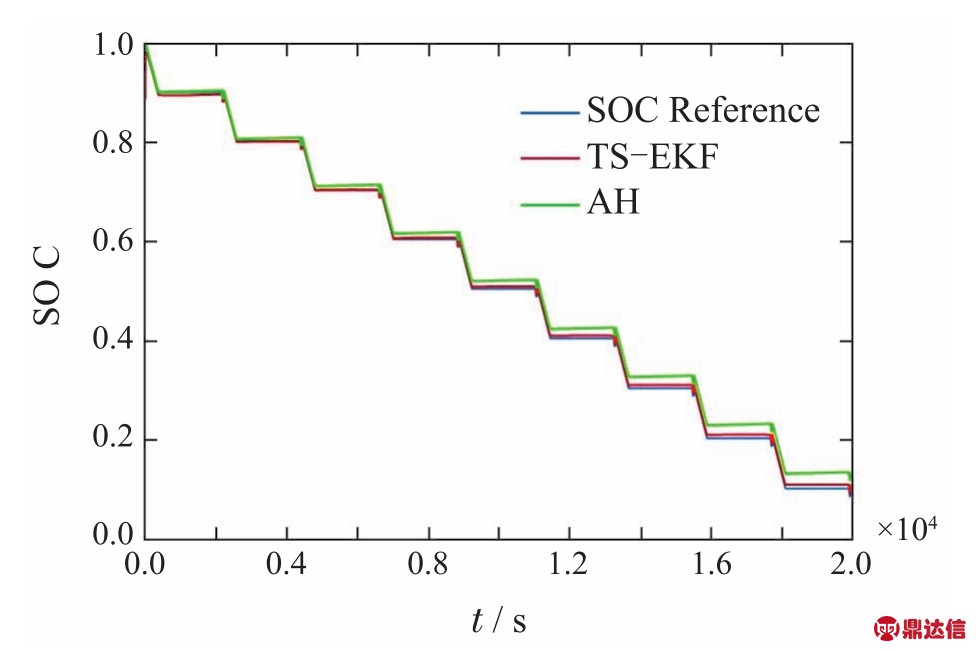
Fig.10 Comparison of TS–EKF and AH estimator
Obviously,the TS–EKF estimator’s performance is better than the AH estimator,because there is no feedback mechanism in the AH estimator,and the accumulated error caused by the input noise cannot be eliminated.Since the TS–EKF estimator has a double correction effect on the unknown noise and the known measurement disturbance,the in fluence of the initial state error on the SOC observation of the lithium battery can be quickly eliminated and the SOC observation value can be finally converged to the true value.
4.2 Comparison of TS–EKF and EKF Estimator
In this experiment,the TS–EKF union estimator is compared with the EKF estimator to verify the TS fuzzy module’s effect on the estimation performance.Test conditions are as follows:the initial value of SOC is 0.8,the surrounding temperature is 20◦C,the constant current is 6 A,under which the lithium battery is discharged continuously until the value of SOC declines to 0.3.
As is shown in Fig.11,the SOC estimation curve obtained by the EKF estimator and the TS–EKF estimator are compared with the SOC reference curve,in which the initial states of the two estimators are both set to[0.35,0,0].It can be seen that the two estimation curves can converge quickly and closely to the SOC reference curve,with only a slight difference in estimation accuracy.
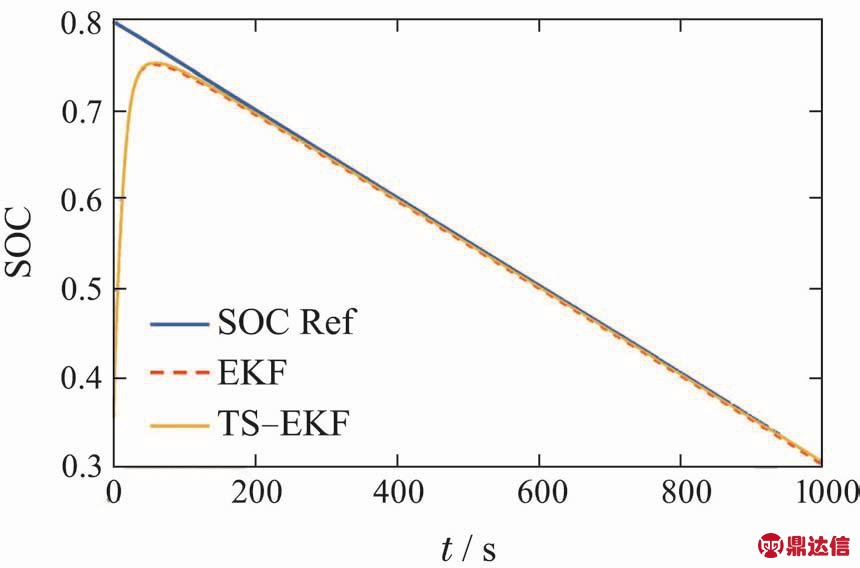
Fig.11 The estimation curves of the TS–EKF and EKF for SOC
The head and end of the estimation error curves in Fig.7 are partially enlarged respectively,as shown in Fig.12 and Fig.13.The average estimation error,mean square error and convergence time for error less than 1%of EKF and TS–EKF union estimator are shown in Table 3.
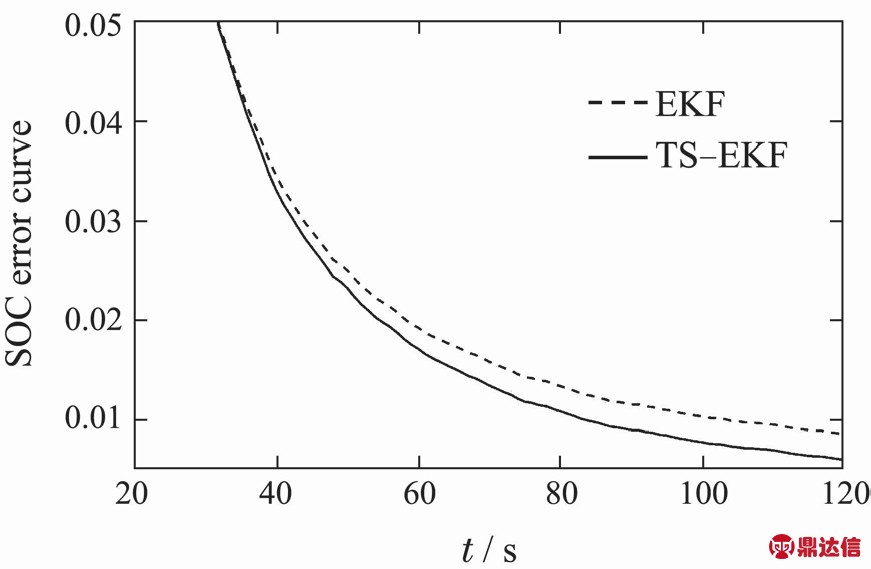
Fig.12 The head of the estimation error curve
In Fig.12,at the head of the SOC error curve,it is shown that the EKF estimator reduces the error of the SOC to less than 0.01 after 105 s,while the error of the TS–EKF estimator is less than 0.01 after 84 s.The convergence speed of the TS–EKF estimator is accelerated by about 25%.In Fig.13,it is shown that the errors of EKF estimator and TS–EKF estimator are about 0.35%and 0.06%respectively at the end of the SOC error curve.The observation error is reduced by 82.9%compared with the original one.From the overall error point of view,the average SOC error of EKF estimator and TS–EKF estimator is 0.77%and 0.47%,respectively.The average error of the latter is 39.0%less than the former.
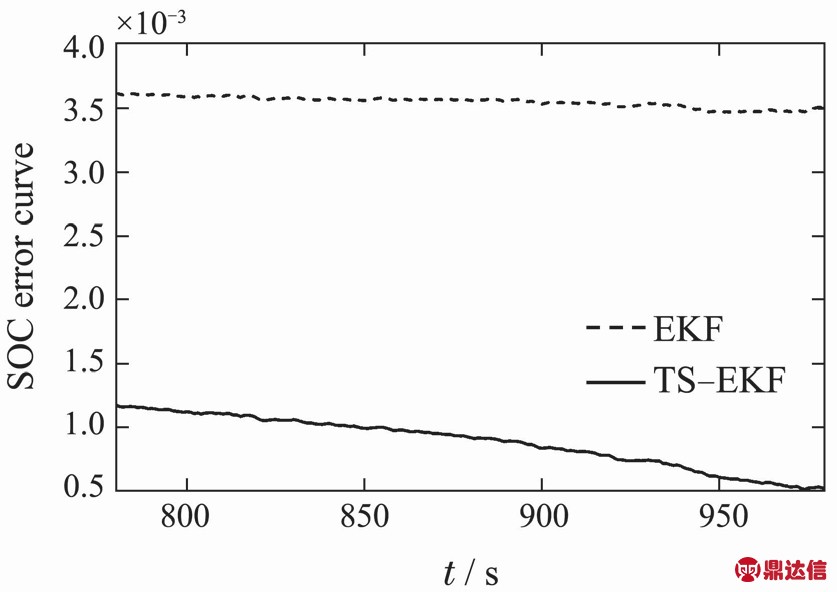
Fig.13 The end of the estimation error curve
Table 3 Error comparison of EKF and TS–EKF
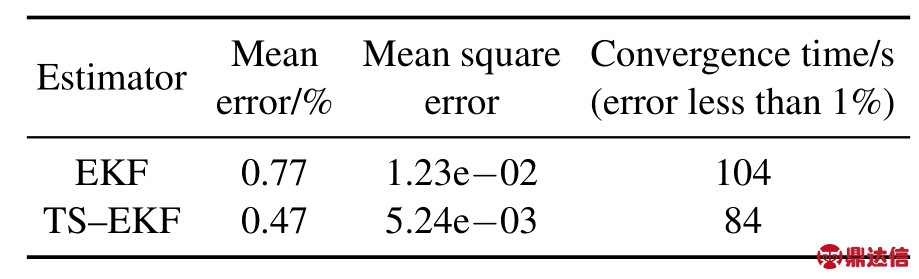
The 2nd-order RC model with variable parameters of battery is used to adjust the relevant parameters with the changes of operating conditions,which makes the model more sensitive to the changes of operating conditions of battery,so as to obtain more accurate SOC observation results.The dual correction effect of TS fuzzy module and EKF module also makes the SOC estimator more robust to random interferencesignals,furtheracceleratestheconvergence speed of TS–EKF union estimator,and improves its SOC observation accuracy significantly.
5 Conclusions
In this paper,based on the 2nd-order RC model with variable parameters,an AH estimator,EKF estimator and TS–EKF union estimator are designed respectively.The TS–EKF union estimator is compared with the AH integral estimator and the EKF estimator through the Simulink platform respectively,and it is verified that the TS–EKF estimator has a better performance in terms of SOC estimation performance.The results show that TS–EKF union estimator can improve the observation accuracy of SOC.
Acknowledgement
I would like to acknowledge CHEN Mingyuan,a graduate student from the school of Automation Huazhong University of Science and Technology,for the work done in the early part of this paper.